Share This Article:
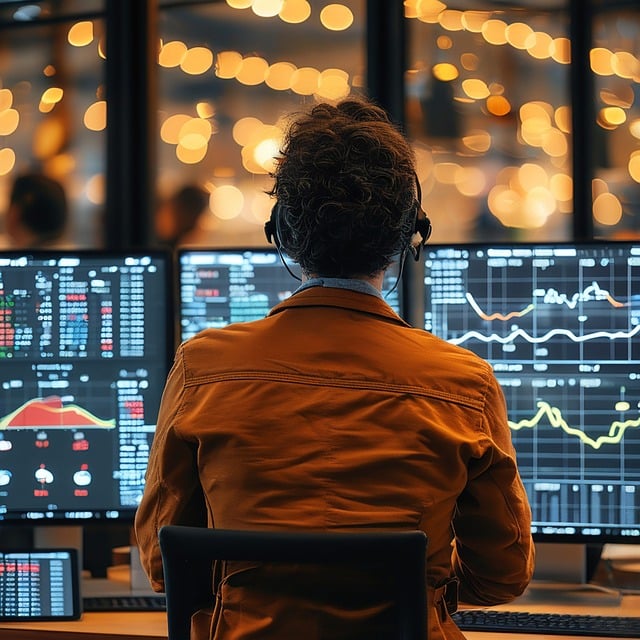
The Impact of Predictive Analytics on Claims Management and Settlement: Revolutionizing Workers’ Compensation for Faster, Smarter Outcomes
19 Sep, 2024 Natalie Torres
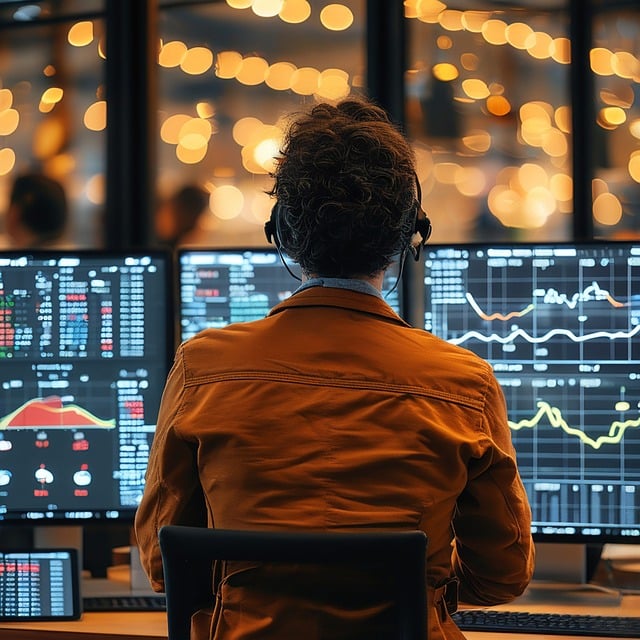
In the ever-evolving landscape of workers’ compensation, insurance, and healthcare, technology is becoming a game-changer in improving efficiency, accuracy, and outcomes. One of the most significant advances shaping this industry today is the application of predictive analytics. Predictive analytics has transformed how claims are managed and settled, providing a proactive approach to handling complex cases and reducing overall costs. Let us explore how predictive analytics is being engaged, what types of data are examined, and how this technology supports more efficient claims management and more efficient settlements.
What are Predictive Analytics?
Predictive analytics uses historical data, statistical algorithms, and machine learning techniques to identify the likelihood of future outcomes. In the context of claims management, predictive analytics examines various data points to assess the risk and potential outcomes of a claim. It allows adjusters and claims professionals to make informed decisions, anticipate issues, and act before problems escalate.
By predicting the likely course of a claim, including potential delays, treatment needs, or litigation, claims professionals can respond proactively to resolve cases faster and with better accuracy.
Types of Predictive Analytics Engaged in Claims Management
Predictive analytics in claims management incorporates several different models to support more efficient decision-making:
1. Risk Scoring Models: These models assign a risk score to each claim based on factors like injury type, employee demographics, and employer history. Claims with high-risk scores might require more resources, proactive monitoring, or early intervention to avoid complications.
2. Fraud Detection Algorithms: By analyzing patterns of behavior, predictive analytics can help identify claims that deviate from the norm and may indicate fraudulent activity. Unusual patterns in medical treatment, employer reporting, or claimant behavior may trigger further investigation, potentially saving time and reducing unnecessary payouts.
3. Medical Treatment Forecasting: These models predict the likely course of medical treatment based on the injury type and historical outcomes for similar cases. This allows claims managers to anticipate treatment costs, recovery timelines, and return-to-work possibilities, helping to manage resources more efficiently.
4. Litigation Propensity Models: Certain claims have a higher likelihood of ending in litigation, which can extend the settlement process and increase costs. Predictive analytics identifies which cases have a higher probability of legal action, allowing for preemptive strategies such as mediation or settlement offers to avoid court.
5. Return-to-Work Models: These models estimate when an injured worker is likely to return to work based on previous cases, the type of injury, and the nature of the job. Accurate return-to-work predictions enable better planning for modified duties or transitional work, reducing the length of claims.
Data Sources Used in Predictive Analytics
The success of predictive analytics depends on the quality and diversity of the data used. Some of the most common types of data examined to support claims management include:
Historical Claims Data: Past claim outcomes are one of the most valuable sources of information. Historical data provides insight into which types of injuries are more likely to result in long-term disability, extensive treatment, or litigation. By analyzing this data, predictive models can estimate the probable course of a new claim based on previous cases with similar circumstances.
Medical Records: Detailed medical data, such as the type of injury, treatment protocols, and recovery timelines, are critical in predicting the future needs of a claim. Medical information helps in assessing the complexity of the case and the likelihood of complications.
Demographic Information: Age, gender, occupation, and geographic location can all influence the outcome of a claim. Predictive analytics can analyze demographic data to determine which factors correlate with longer recovery times or increased likelihood of litigation.
Employer Data: The history of the employer, including safety records, claim frequency, and return-to-work programs, can influence the risk score of a claim. Employers with robust safety programs and return-to-work initiatives may have claims that resolve more quickly.
External Data Sources: Public databases, economic trends, and even weather patterns can provide additional context for claims. For example, claims for injuries caused by extreme weather conditions might require different handling than standard workplace incidents.
How Predictive Analytics Supports Claims Management
Predictive analytics plays a critical role in streamlining claims management processes, leading to more efficient and effective outcomes. Here are some of the specific ways predictive analytics improves claims management:
1. Early Identification of High-Risk Claims: By analyzing a claim’s characteristics as soon as it is filed, predictive models can flag high-risk claims for additional attention. This allows adjusters to allocate resources more effectively, addressing potential issues before they escalate. Early identification of complex cases reduces delays in treatment and minimizes the chance of long-term complications.
2. Tailored Claim Handling: Not all claims are the same, and predictive analytics helps segment claims based on their unique characteristics. High-risk or complex claims can be routed to experienced adjusters, while simpler claims can be handled through more automated processes. This ensures that each claim receives the appropriate level of attention, optimizing efficiency.
3. Improved Medical Case Management: Predictive analytics can forecast the likely medical treatment path and associated costs for an injured worker. This allows claims professionals to work more closely with healthcare providers to ensure timely, cost-effective care. By predicting when medical care may deviate from the norm, adjusters can intervene earlier to manage costs and facilitate faster recovery.
4. Proactive Return-to-Work Planning: Predicting the likely return-to-work date enables employers and claims managers to plan for modified or transitional work arrangements. This reduces the amount of time an employee spends out of work, leading to faster claim closures and lower overall costs.
5. Optimized Resource Allocation: Predictive analytics helps claims professionals allocate resources more efficiently. High-risk claims receive more attention, while low-risk claims can be handled through streamlined processes. This ensures that staff time and effort are focused on cases where they can have the greatest impact.
How Predictive Analytics Expedites Claims Settlement
One of the most tangible benefits of predictive analytics is its ability to accelerate the settlement of claims. Here’s how:
1. Faster Decision-Making: Predictive models provide real-time insights into the likely trajectory of a claim, allowing adjusters to make faster decisions about how to handle each case. This reduces the time spent on manual analysis and enables more proactive case management.
2. Early Intervention: By identifying high-risk claims early, predictive analytics enables early intervention strategies, such as additional medical resources or settlement offers. Early intervention reduces the risk of complications, minimizes litigation, and accelerates settlement.
3. Avoidance of Litigation: Claims with a high likelihood of litigation can be handled more strategically, with mediation or settlement offers made before legal action is taken. Avoiding litigation speeds up the resolution process and reduces legal expenses.
4. Faster Return-to-Work: Predictive analytics helps plan for early return-to-work opportunities, reducing the length of claims and speeding up the overall settlement process.
5. Streamlined Processes: Low-risk, straightforward claims can be processed more quickly using automation and standardized workflows, freeing up resources for more complex cases. This leads to faster settlements for simpler claims.
Predictive analytics is transforming the world of claims management and settlement, particularly in the workers’ compensation, insurance, and healthcare sectors. By leveraging data-driven insights, claims professionals can manage cases more efficiently, reduce costs, and settle claims faster. From early identification of high-risk claims to proactive medical management and return-to-work planning, predictive analytics offers a proactive, intelligent approach that benefits both employers and employees alike.
As technology continues to advance, the role of predictive analytics in claims management will only grow, making it an indispensable tool for professionals seeking to optimize outcomes in a competitive industry.
AI california case management case management focus claims compensability compliance courts covid do you know the rule ethics exclusive remedy florida glossary check health care Healthcare hr homeroom insurance iowa leadership medical medicare NCCI new jersey new york ohio opioids osha pennsylvania Safety simply research state info technology texas violence washington WDYT west virginia what do you think women's history month workers' comp 101 workers' recovery workers' compensation contact information Workplace Safety Workplace Violence
Read Also
- Sep 13, 2024
- Sarah Scott Jill Falb
- Sep 12, 2024
- John Alchemy Avery Steffen
About The Author
About The Author
- Natalie Torres
More by This Author
Read More
- Sep 13, 2024
- Sarah Scott Jill Falb
- Sep 12, 2024
- John Alchemy Avery Steffen
- Sep 12, 2024
- NCCI
- Sep 11, 2024
- Lainey Haynes
- Sep 10, 2024
- John Alchemy Avery Steffen
- Sep 02, 2024
- Nicole Usher