Share This Article:
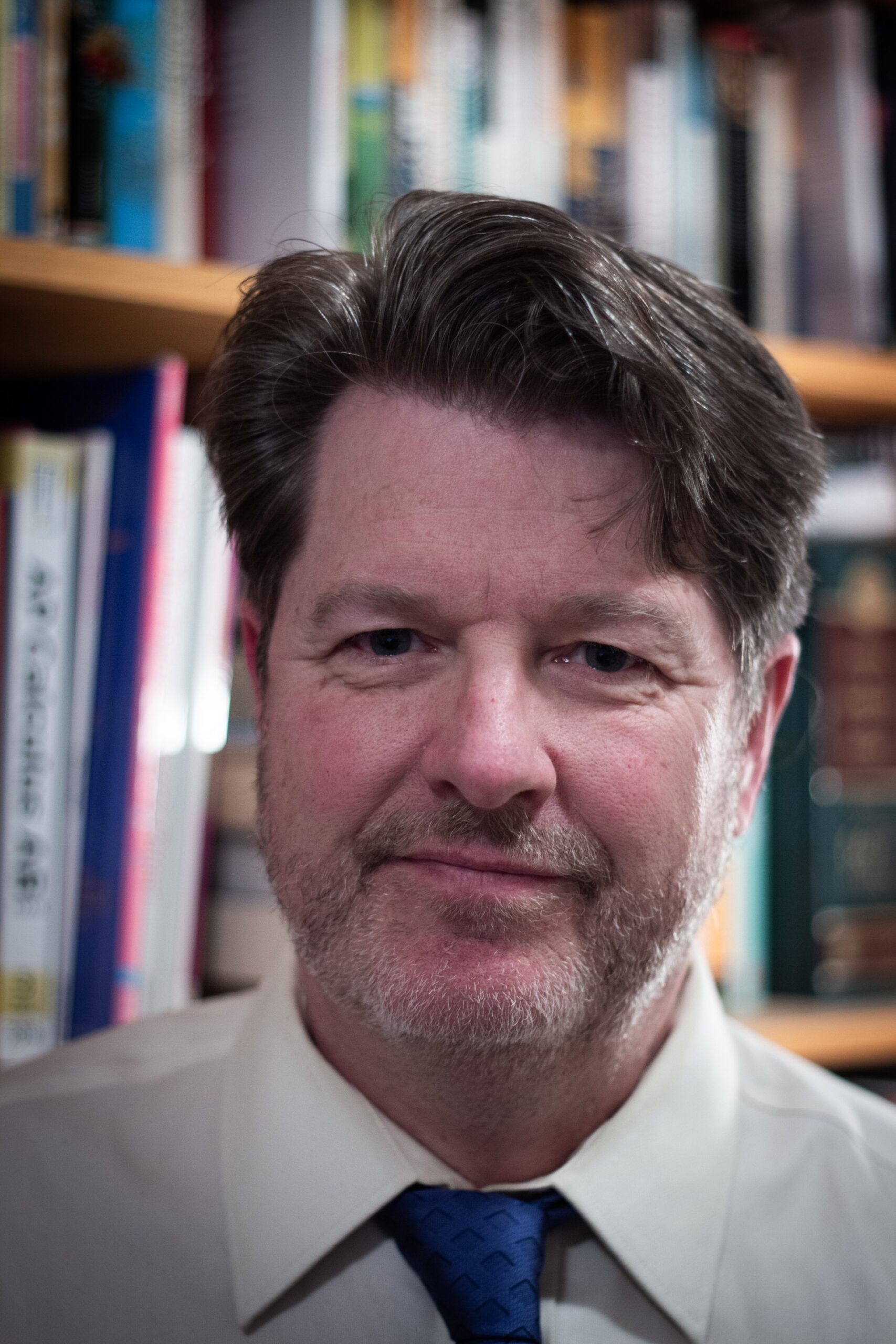
Building the Foundation: High-Accuracy Databases for AI in Workers’ Compensation
09 Oct, 2024 John Alchemy
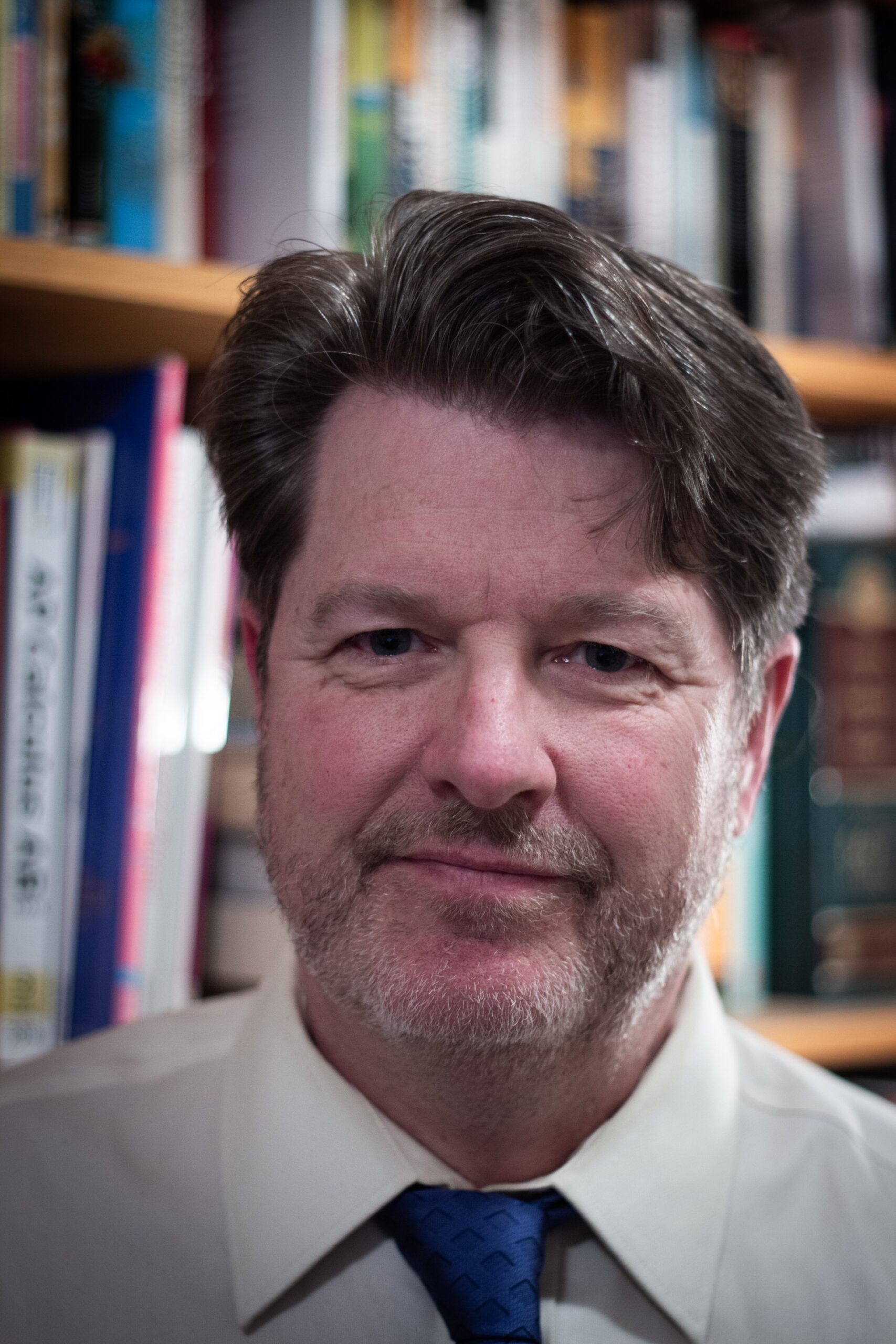
Artificial Intelligence (AI) is revolutionizing industries by enabling automated, efficient, and data-driven decision-making processes. However, AI’s effectiveness relies heavily on the quality of the data it processes. In sectors like workers’ compensation, which deal with complex medical and legal variables, high-accuracy databases are essential to ensure that AI delivers meaningful and reliable results. Standardization and rigorous vetting of data are necessary to improve AI’s accuracy and consistency, particularly in processes like impairment rating, where even minor inaccuracies can have significant implications for both employers and injured workers on return to work, administrative costs, and litigation.
How do you prepare a database to deliver accurate insights via generative AI (GenAI) queries? It’s a delicate exercise in standardization, vetting data, expert oversight, and integrating multiple data sets. Lots of work needs to be performed on the underlying pool of data that AI is tasked with synthesizing, and it must be done so with respect to privacy and security concerns.
The Importance of Standardization
One of the foundational elements of building a high-accuracy database for AI in workers’ compensation is the practice of standardizing the data that goes into the system. Without standardized inputs, AI cannot produce consistent or meaningful outputs. In workers’ compensation, where impairment ratings can vary widely between different providers or insurance carriers, standardization ensures that every report is measured against the same criteria.
For example, a doctor’s report may classify arthritis found in an X-ray as “not otherwise specified.” However, this ambiguity needs to be addressed for the purposes of impairment rating. In this case, the database should classify the arthritis as “at least mild” to ensure consistency in scoring. Similarly, when assessing “shoulder girdle weakness,” if the report doesn’t specify the grade, the database may select to rate this conservatively as a 4 out of 5 in motor strength testing. This approach ensures that AI, when generating reports or making predictions, is working with reliable and uniform data.
Data Vetting: Ensuring Accuracy Before Input
Data standardization alone isn’t enough. A high-accuracy database must also have stringent vetting processes to ensure that the data being entered is both accurate and relevant. In the case of workers’ compensation impairment rating, this means that data collected from medical reports must be scrutinized by experts who understand both the medical context and the AI’s computational processes and tendencies.
Before data is committed to the database, human experts must validate the inputs, verify that the proper standardization procedures were followed, and ensure that the results generated by AI models are accurate. Experts must understand not just the medical terminology, but also the technicalities of the variable thread analytic computations (VTAC), which overlay data points to compute impairment scores. After standardizing a doctor’s report, for instance, experts must check that the AI-generated impairment rating is both accurate and consistent with medical guidelines before finalizing the entry in the database.
This level of vetting is resource-intensive but crucial for maintaining a high-accuracy database. As the database grows, the returns on these investments become clear—more accurate AI models lead to faster settlements, more consistent impairment ratings, and a significant reduction in disputes over workers’ compensation claims.
Integrating Multiple Data Sets
Another challenge in building high-accuracy databases for AI usage in workers’ compensation involves integrating multiple data sets from various sources. The workers’ compensation industry uses a range of rating systems and guidelines, such as the AMA Guides to Permanent Impairment (The Guides) 4th, 5th, and 6th editions, all of which may yield different impairment ratings for the same condition. Merging these diverse data sets into a cohesive, standardized system is essential for the AI engine to produce reliable outputs and take advantage of historical data sets with different computational backgrounds.
For example, a workers’ compensation claim that follows The AMA Guides 5th Edition might generate a different impairment rating than one based on the 6th edition. Without proper integration, the AI would struggle to make meaningful comparisons or predictions across claims. Therefore, the database must account for these differences and provide standardized conversions between the editions, allowing AI to make accurate predictions regardless of which system the original report follows. These new streamlined standards also need to be carefully documented; versions and dates of updates become exceedingly important if the AI algorithm needs to be adjusted or investigated should system errors emerge later in the process.
Additionally, historical data must be carefully handled to ensure accuracy. Rounding methods and mathematical computations must be consistent across different cases. When summarizing a final claim value, experts should ensure that digits are carried out to the appropriate levels, avoiding discrepancies that could affect the final outcome and ultimately cost outcomes.
Data Privacy, Security, and PHI Concerns
Workers’ compensation databases must also address concerns about privacy and security, particularly when dealing with personal health information (PHI). Medical records often contain sensitive data, such as psychiatric evaluations or diagnoses of infectious diseases, which must be handled with care to comply with privacy regulations like HIPAA.
Moreover, PHI often has an expiration date, meaning that records must be purged after a certain period. In the context of high-accuracy databases, this presents a challenge: How do you maintain a robust and reliable reference set while complying with privacy regulations? One solution is to anonymize and sanitize data before entering it into the database, allowing it to remain in the system without posing a security risk.
Anonymization and encryption processes are not just beneficial for maintaining the integrity of the data but also for reducing the risks and costs associated with potential data breaches. For example, if a database is breached, the lack of personally identifiable information (PII) limits the exposure and liability faced by the organization.
Balancing Data Privacy with Database Integrity
While anonymizing data is crucial, it also presents the risk of losing important context that could affect the accuracy of AI models. Striking a balance between privacy and comprehensiveness of the data is an ongoing challenge. It requires collaboration between the medical team, data scientists, and security experts.
Moreover, bias and prejudice can creep into AI models if the data is not handled correctly. For example, if the algorithm assigns higher impairment ratings to certain demographics based on incomplete or biased data, it could unfairly impact the outcomes for certain groups of injured workers, geographic regions, or job classifications. Preventing these biases requires continuous oversight and regular auditing of both the data and the algorithms.
Cost vs. Value: Is It Worth the Investment?
Building and maintaining a high-accuracy database is a costly endeavor. The need for highly skilled experts, rigorous vetting processes, and advanced security measures can make this an expensive proposition for many organizations. However, the long-term value of such an investment far outweighs the upfront costs.
By investing in a high-accuracy database, organizations can achieve significant future savings. Accurate impairment ratings reduce the time to settlement, minimizing legal disputes and administrative costs. Additionally, reliable data allows AI to make more informed decisions, which in turn leads to better resource allocation for medical treatments and return-to-work programs.
A high-accuracy database can also serve as a competitive advantage for companies that invest in it. As AI continues to evolve and become more integrated into workers’ compensation processes, organizations with superior data sets will be able to offer more efficient, accurate, and cost-effective services than their competitors.
The Effort Pays Off
Building a high-accuracy database for AI in workers’ compensation is a complex but essential task. The success of AI models in this space depends on the quality, accuracy, and standardization of the data they process. Addressing these challenges—data vetting, the integration of multiple data sets, and privacy concerns—is critical to building a reliable database that can serve as the foundation for AI-driven improvements in workers’ compensation.
While the cost of developing and maintaining such a database may be high, the long-term benefits—faster settlements, more consistent impairment ratings, and reduced legal disputes—make it a worthwhile investment. As AI continues to transform the industry, companies that invest in high-accuracy databases will have a distinct competitive advantage, positioning themselves as leaders in the field of workers’ compensation.
california case management case management focus claims compensability compliance courts covid do you know the rule emotions exclusive remedy florida FMLA glossary check health care Healthcare hr homeroom insurance insurers iowa kentucky leadership medical NCCI new jersey new york ohio osha pennsylvania roadmap Safety simply research state info technology texas violence WDYT west virginia what do you think women's history month workcompcollege workers' comp 101 workers' recovery Workplace Safety Workplace Violence
Read Also
- Feb 17, 2025
- Lainey Haynes
- Feb 12, 2025
- NCCI
About The Author
About The Author
- John Alchemy
Read More
- Feb 17, 2025
- Lainey Haynes
- Feb 12, 2025
- NCCI
- Feb 11, 2025
- NCCI
- Feb 05, 2025
- NCCI
- Jan 30, 2025
- Shawn Deane
- Jan 29, 2025
- Donita Stacker