Share This Article:
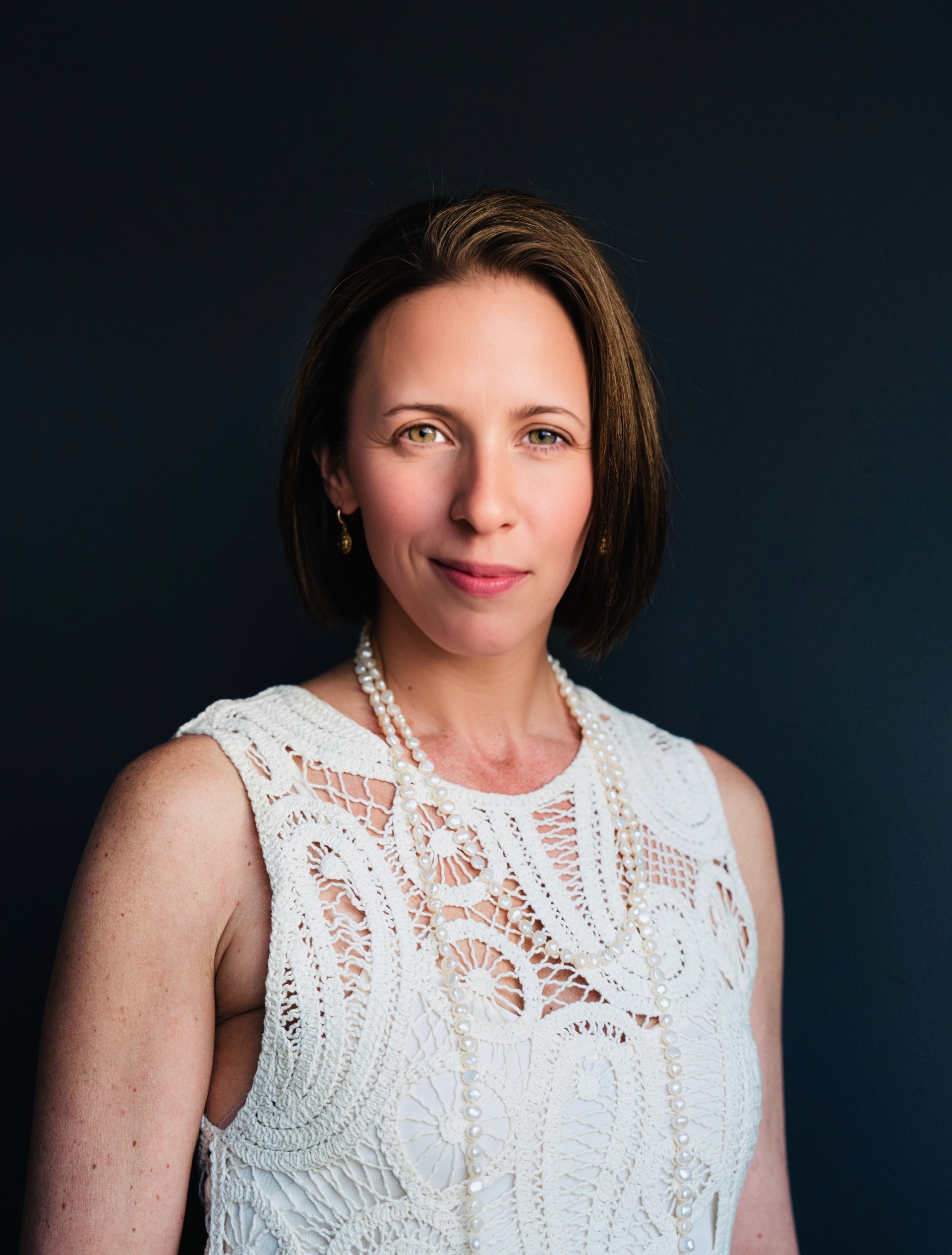
Data-Driven Insights: Enhancing Workplace Safety Through Predictive Analytics
09 Oct, 2024 Natalie Torres
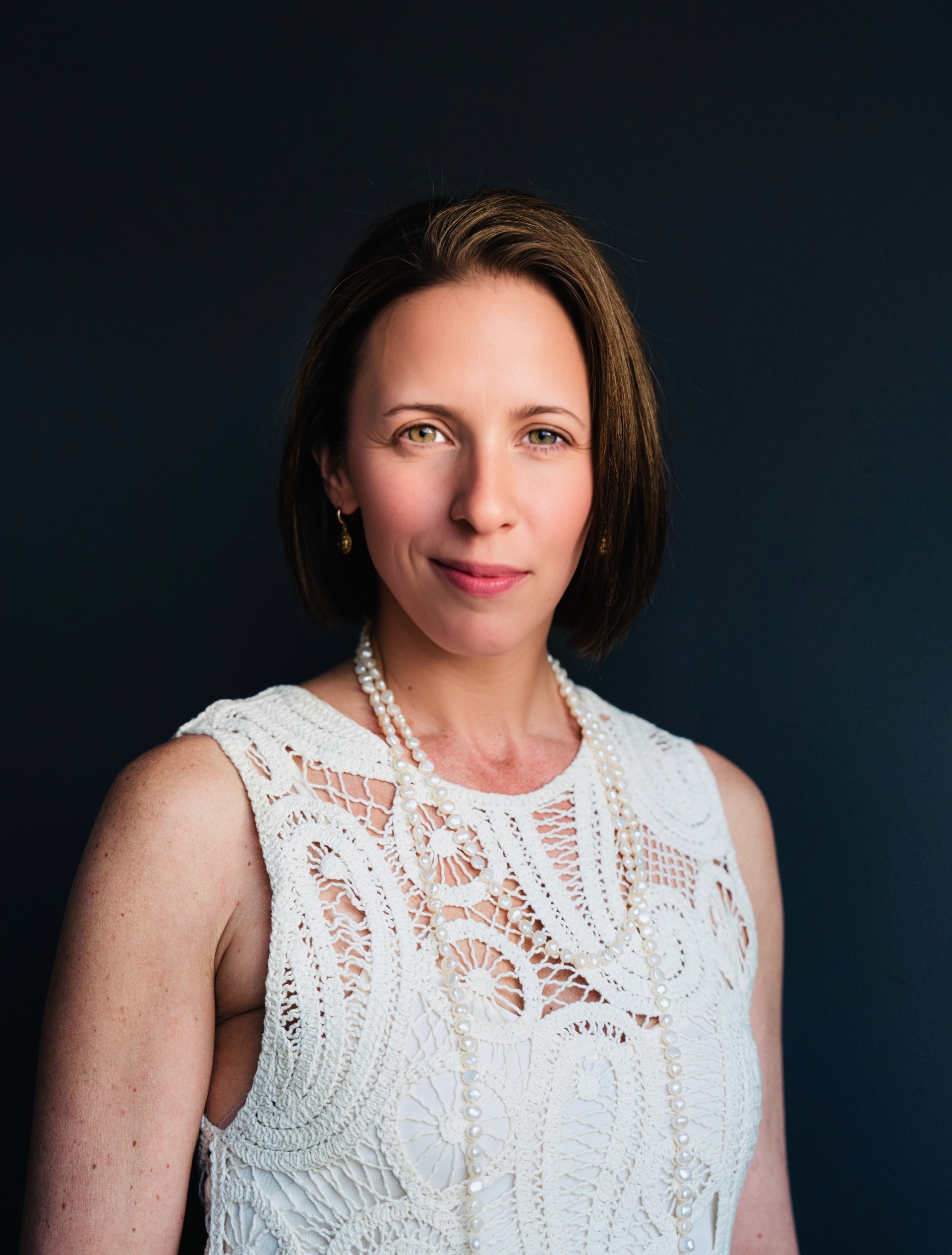
In an era where the velocity of business is accelerating at an unprecedented pace, workplace safety has evolved from being a simple compliance obligation to becoming a strategic imperative. For organizations that prioritize sustainable growth, the integration of predictive analytics in safety protocols represents a seismic shift, offering not only insights into preventing workplace injuries but also the opportunity to drive efficiency and reduce costs across the board. For stakeholders in workers’ compensation, healthcare, and insurance, leveraging data analytics for injury risk prediction is an innovation that elevates the conversation about safety management to new heights.
At its core, predictive analytics employs data, statistical algorithms, and machine learning techniques to identify the likelihood of future outcomes based on historical data. In the context of workplace safety, this means using the vast troves of data now available—from employee demographics and historical injury reports to environmental conditions and operational metrics—to proactively forecast potential safety risks. The outcome? Organizations are better equipped to anticipate and address hazards before they materialize into costly and often debilitating injuries.
The Role of Predictive Analytics in Risk Prevention
Predictive analytics has proven to be a powerful tool in numerous industries, but its application in workplace safety is particularly compelling. Traditional safety protocols, which often rely on post-incident analysis, are inherently reactive. In contrast, predictive analytics enables organizations to adopt a proactive stance, empowering safety officers, risk managers, and healthcare providers to pinpoint areas of concern before an accident occurs. This foresight can significantly reduce injury frequency and severity, translating to lower claims and, ultimately, reduced insurance premiums.
For instance, predictive models can identify patterns in injury data, such as correlations between shift lengths, worker fatigue, and certain types of injuries. It can also analyze environmental factors, including temperature or noise levels, to predict when and where accidents are most likely to occur. By incorporating these insights into daily operations, companies can optimize work schedules, introduce targeted training, and implement environmental adjustments that diminish the likelihood of accidents.
Predictive analytics offers the potential to revolutionize employee health monitoring. Wearable devices, which track physiological data such as heart rate and body temperature, provide real-time insights into an individual’s physical condition. When integrated with predictive algorithms, this data can alert managers when an employee is approaching the threshold of overexertion or is at risk of experiencing heat stress, thereby averting injuries before they occur.
Data Sources Powering Predictive Models
A key component of building effective predictive models is access to comprehensive and high-quality data. Organizations looking to implement injury risk prediction systems must draw from a variety of data sources to ensure the robustness of their models. Some critical sources include:
Historical Claims Data: Workers’ compensation claims data provides a wealth of information about previous incidents, including the nature of injuries, contributing factors, and recovery timelines. This data allows predictive models to recognize patterns in injury types and identify high-risk areas within an organization.
Workforce Demographics: Information related to employee age, job tenure, skill level, and physical fitness is crucial in understanding individual risk factors. Different demographic groups may be more susceptible to certain injuries, and incorporating this data into predictive models enables a more nuanced risk analysis.
Environmental and Operational Data: Factors such as workplace temperature, lighting, noise levels, and machinery usage can all contribute to injury risks. By tracking these environmental conditions in real-time, organizations can identify unsafe working environments and take preventive action before accidents occur.
Health and Wellness Data: With the rise of wearables and health monitoring technologies, organizations can collect physiological data that provides insights into an employee’s well-being. Monitoring heart rate, sleep patterns, and physical exertion levels can help predict when an individual is at risk of injury due to fatigue or other health-related factors.
Behavioral and Psychological Data: Emerging research shows that mental health and cognitive states—such as stress, distraction, or anxiety—play a significant role in injury risk. By integrating behavioral and psychological data into predictive models, companies can identify when an employee’s mental state might impair their ability to work safely.
The Impact on Workers’ Compensation and Healthcare
For executives in workers’ compensation, healthcare, and insurance, the predictive power of data analytics represents a unique value proposition that reshapes traditional approaches to injury management. In the workers’ compensation space, where costs related to workplace injuries and lost productivity are substantial, predictive analytics can lead to a marked decrease in claims frequency and severity. Organizations that leverage predictive models are not only better equipped to prevent injuries, but also more capable of managing claims when they do arise. By identifying high-risk employees and environments, companies can implement targeted interventions that reduce the number of injuries and expedite return-to-work processes.
Healthcare providers, particularly those focused on occupational health, also stand to benefit from predictive analytics. By integrating data on patient health, treatment outcomes, and recovery trajectories into predictive models, healthcare organizations can enhance patient care while optimizing resource allocation. In the context of workplace injuries, predictive analytics allows healthcare professionals to anticipate which treatments are most likely to succeed for specific injury types, streamlining the path to recovery and minimizing time away from work.
Insurance carriers, too, can capitalize on the predictive capabilities of data analytics. With a deeper understanding of an organization’s risk profile, insurers can offer more precise and competitive pricing. Moreover, predictive analytics empowers insurance companies to engage in risk mitigation efforts proactively, working alongside policyholders to improve workplace safety before claims ever arise.
Overcoming Barriers to Adoption
Despite the clear advantages, the adoption of predictive analytics in workplace safety still faces several challenges. The most significant hurdle is often the availability and quality of data. Many organizations struggle with data silos, where critical information is fragmented across different departments or systems, making it difficult to build comprehensive predictive models. Furthermore, the accuracy of predictions depends heavily on the quality of the data being used. Incomplete or erroneous data can lead to flawed predictions, undermining the effectiveness of injury prevention efforts.
Another challenge is the potential resistance to change within organizations. Implementing predictive analytics requires a shift in mindset from reactive safety management to a more proactive, data-driven approach. This transformation necessitates buy-in from multiple stakeholders, including executives, safety officers, and front-line employees, all of whom must understand the value that predictive analytics brings to the table.
Finally, data privacy and security concerns must be addressed, particularly when dealing with sensitive health and personal information. Organizations need to ensure that robust safeguards are in place to protect employee data, adhering to regulatory requirements while maintaining trust with their workforce.
As workplace environments become increasingly complex, the need for innovative approaches to injury prevention has never been more pressing. Predictive analytics offers a powerful solution, enabling organizations to move beyond traditional safety protocols and embrace a proactive, data-driven strategy. By harnessing the power of data, companies can not only predict and prevent injuries but also reduce costs, improve employee well-being, and enhance operational efficiency.
For executives in workers’ compensation, healthcare, and insurance, the integration of predictive analytics into safety management is no longer a futuristic concept—it is a business imperative. Organizations that invest in this technology will position themselves at the forefront of workplace safety innovation, ensuring a safer and more resilient future for their employees and stakeholders.
AI california case management case management focus claims compensability compliance courts covid do you know the rule emotions exclusive remedy florida FMLA glossary check Healthcare health care hr homeroom insurance insurers iowa leadership medical NCCI new jersey new york ohio osha pennsylvania roadmap Safety state info technology texas violence WDYT west virginia what do you think women's history women's history month workcompcollege workers' comp 101 workers' recovery Workplace Safety Workplace Violence
Read Also
About The Author
About The Author
- Natalie Torres
More by This Author
Read More
- Apr 01, 2025
- NCCI
- Mar 30, 2025
- Kim Radcliffe
- Mar 29, 2025
- Natalie Torres
- Mar 19, 2025
- NCCI
- Mar 16, 2025
- Derek Goff
- Mar 13, 2025
- Rayford Taylor